VECO: Cross-lingual Pre-training
VECO stands for “Variable and Flexible Cross-lingual Pre-training” which is a pre-training approach for cross-lingual language models that uses “Cross-Attention Masked Language Modeling” (CA-MLM) objective. VECO was proposed by Alibaba Group in 2020 and published in their “VECO: Variable and Flexible Cross-lingual Pre-training for Language Understanding and Generation” paper. The official code for this paper can be found on Alibaba’s official GitHub repository: alibaba/VECO.
CA-MLM Pre-training
The special thing about VECO pre-training is that it plugs a cross-attention module into the Transformer architecture to explicitly build the cross-relation between languages when pre-training using the Cross-Attention Masked Language Modeling MLM (CA-MLM) objective. The following figure shows the difference between MLM pre-training without plugging cross-attention module (left) and with plugging the cross-attention module (right):
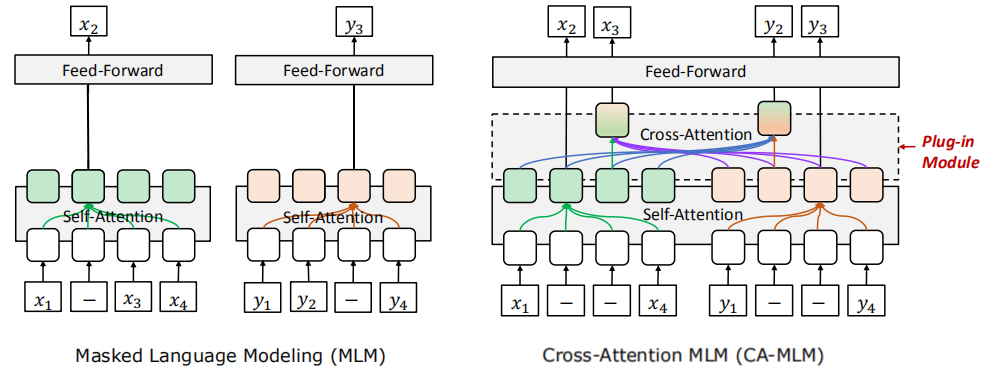
Note:
MLM here is different than MLM objective proposed in the BERT paper. As we can see, here MLM is applied on both encoder and decoder.
Given a pair of input $\left( x,\ y \right)$ and its MLM corrupted version $\left( \widehat{x},\ \widehat{y} \right)$, the model tries to minimize the following loss:
\[\mathcal{L}\left( x,\ y \right) = - \log P\left( x \middle| \widehat{x};\ \theta_{s} \right) - \log P\left( x \middle| \widehat{y},\ \widehat{x};\ \theta_{s},\ \theta_{c} \right)\] \[\ \ \ \ \ \ \ \ \ \ \ \ \ \ \ \ \ \ - \log P\left( y \middle| \widehat{y};\ \theta_{s} \right) - \log P\left( y \middle| \widehat{x},\ \widehat{y};\ \theta_{s},\ \theta_{c} \right)\]Where $\theta_{s}$ and $\theta_{c}$ are the parameters of self-attention and cross-attention modules respectively.
Note:
In case of monolingual data, CA-MLM uses two adjacent sentences as the $\left( x,\ y \right)$ pair.
Cross-Attention Module
As said before, the VECO pre-training extends the Transformer architecture and plugs a cross-attention module in each layer. Now, each layer has three modules: self-attention module, a plug-and-play cross-attention module, and a feed-forward linear module. Both self-attention and cross-attention modules are based on the multi-head attention mechanism.
An attention function can be described as mapping a query $Q$ and a set of key-value $K,V$ pairs to an output. For the self-attention module, all the queries, keys and values are the same representations from the previous layer. Specifically, for the $l^{\text{th}}$ layer, the output of a self-attention head $A_{l}^{s}$ is computed via:
\[Q = H^{l - 1}W_{l}^{Q},\ \ \ K = H^{l - 1}W_{l}^{K},\ \ \ V = H^{l - 1}W_{l}^{V}\] \[A_{l}^{s} = \text{softmax}\left( \frac{QK^{T}}{\sqrt{d_{k}}} \right)V\]Where $H^{l - 1}$ is the output of the previous layer, and $W_{i}^{Q},W_{i}^{K},W_{i}^{V}$ are learned parameter matrices of the self-attention.
For the cross-attention module, the queries come from the previous layer, and the keys and values come from the last layer’s representations of paired input. Specifically, for the $l^{\text{th}}$ layer, the output of a cross-attention head $A_{l}^{c}$ is computed via:
\[Q = S^{l - 1}U_{l}^{Q},\ \ \ K = H^{L}U_{l}^{K},\ \ \ V = H^{L}U_{l}^{V}\] \[A_{l}^{c} = \text{softmax}\left( \frac{QK^{T}}{\sqrt{d_{k}}} \right)V\]Where $S^{l - 1}$ is the output of the previous layer, $U_{i}^{Q},U_{i}^{K},\ U_{i}^{V}$ are learned parameter matrices of the cross-attention, and finally $H^{L}$ is the output of the last layer.
Note:
There are two types of contextualized vector representation:\[P\left( x \middle| \widehat{x} \right) = \text{softmax}\left( f\left( H_{x}^{L} \right) \right),\ \ \ \ \ P\left( y \middle| \widehat{y} \right) = \text{softmax}\left( f\left( H_{y}^{L} \right) \right)\]
- $H$: Which depends on the masked sequence:
\[P\left( x \middle| \widehat{x},\ \widehat{y} \right) = \text{softmax}\left( f\left( S_{x}^{L} \right) \right),\ \ \ \ \ P\left( y \middle| \widehat{x},\ \widehat{y} \right) = \text{softmax}\left( f\left( S_{y}^{L} \right) \right)\]
- $S$: Which depends on the masked paired sequence:
Furthermore, this cross-attention module can be plugged-in or plugged-out on-demand which makes it very suitable for both cross-lingual language understanding tasks and generation tasks as we are going to see next.
Regrading cross-lingual understanding tasks on the XTREME benchmark, VECO ranks first at the leaderboard at the submission deadline. Regrading cross-lingual generation tasks such as machine translation, VECO outperforms existing state-of-the-art models by 1∼2 BLEU score.
Fine-tuning
As illustrated in the following figure, VECO is very flexible when fine-tuning on various downstream tasks due to the plug-and-play cross-attention module. It can be used for initializing the encoder-only Transformer for natural language understanding (NLU) tasks and encoder-decoder Transformer for natural language generation (NLG) tasks.
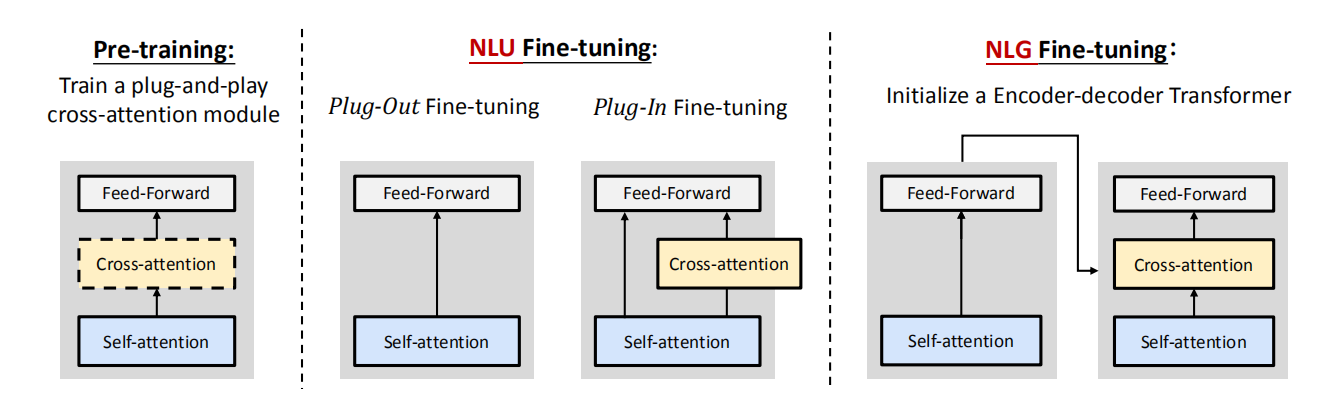
During NLU fine-tuning, there are two approaches that we can choose from:
-
Plug-out fine-tuning: is to unplug the cross-attention module from the pre-trained model which makes the model very similar to mBERT.
-
Plug-in fine-tuning: is to plug the cross-attention module into the fine-tuned model. This can be used if the other language y is available in the downstream task. In this approach, the two representations $\left\lbrack H_{x}^{L};S_{x}^{L} \right\rbrack$ are concatenated to predict the label of $x$ and $\left\lbrack H_{y}^{L};S_{y}^{L} \right\rbrack$ are concatenated to predict the label of $y$.
Experiments & Results
They collected monolingual and bilingual corpus covering 50 languages. For monolingual training datasets, they extracted 1.36TB data in 50 languages from the CommonCrawl Corpus, which contains 6.5G sentences and 0.4G documents. The following table has statistics for all 50 languages monolingual data where the values are in million:
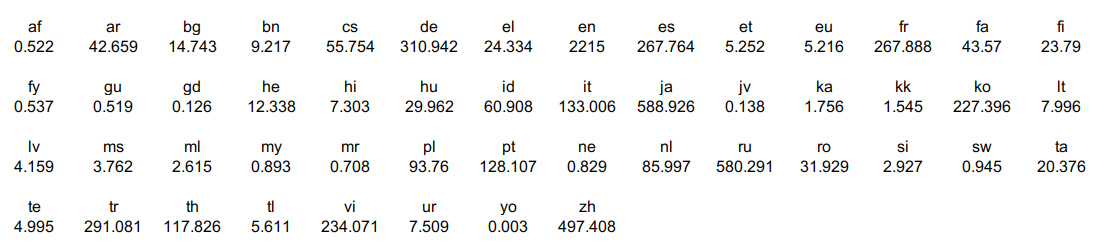
They up/down-sample the monolingual text like XLM from each language with a smoothing parameter $\alpha = 0.5$ where $D_{l}$ is the number of sentences in language $l$.
\[p_{l} = \left( \frac{D_{l}}{\sum_{k}^{}D_{k}} \right)^{\alpha}\]For bilingual data, they extracted 6.4G parallel sentences across 50 languages from the OPUS website.
In this paper, they used pre-trained two VECO models following the XLM-R model. The large one is a 24-layer model with 1024 embedding/hidden size and 4096 feed-forward size, while the small one is 6 layers with 768 embedding/hidden size and 3072 feed-forward size. Also, they used the same 250K vocabulary that was used by XLM-R . The full list of the model can be seen in the following table:
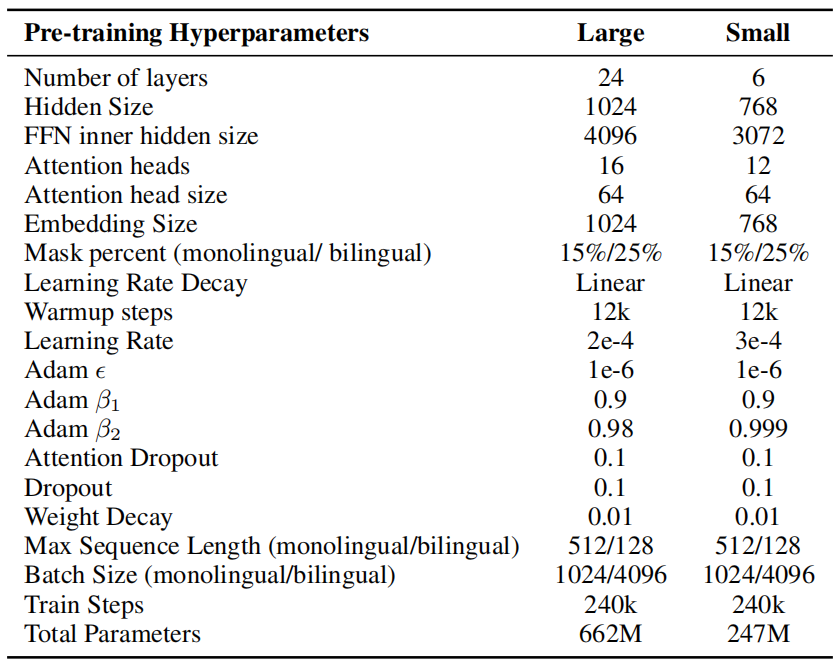
The following table shows a comparison between VECO and other baselines:

When fine-tuning on natural language understanding tasks, VECO outperforms previous cross-lingual models on all datasets of the XTREME benchmark:
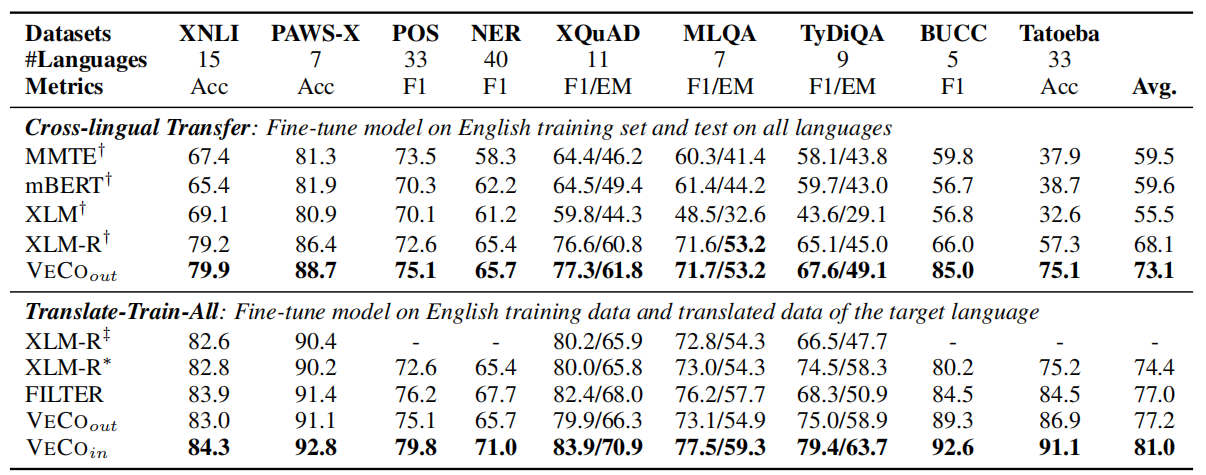
When fine-tuning on natural language generation tasks (machine translation), VECO outperforms the randomly initialized same-sized Transformer baseline by 2.3 BLEU points. Moreover, it even beats the (randomly initialized) state-of-the-art Deep-Transformer, which is three times deep as VECO.
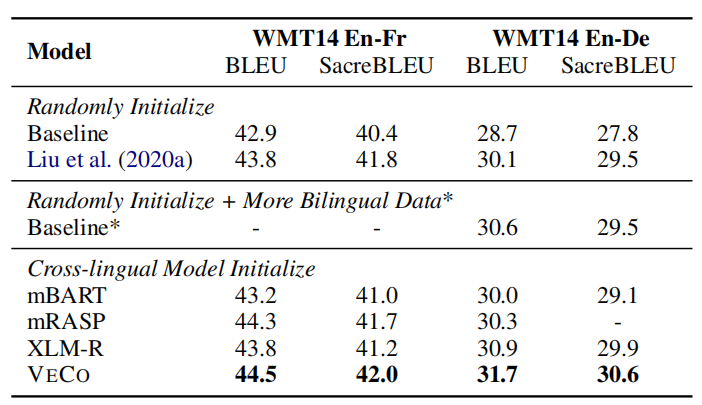
And to investigate where the improvement in VECO comes from, they trained XLM, mBART and VECO model from scratch using the same datasets and parameter settings where all of them were pre-trained on MLM and TLM tasks and fine-tuned on XNLI downstream task and machine translation De-En pair from the IWSLT14 dataset. The results are shown in the following table:
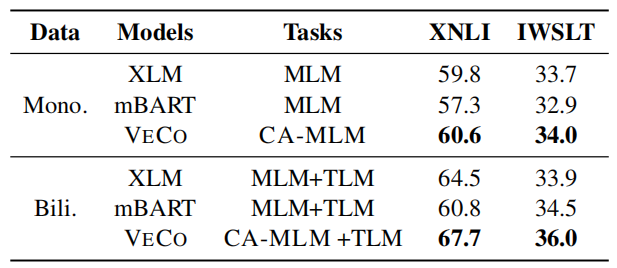
The previous table shows that:
-
When pre-training on monolingual data only, VECO outperforms XLM by 0.8 points on the XNLI dataset and 0.3 BLEU scores on the IWSLT dataset which suggests that CA-MLM can still benefit from adjacent sentences in monolingual corpus. Remember that CA-MLM uses adjacent sentences as the $\left( x,y \right)$ pair.
-
When pre-training on both monolingual and bilingual data, VECO achieved a larger improvement compared to XLM, with 3.2 and 2.1 points improvement on the two datasets, respectively. It reveals that CA-MLM objective of VECO can better utilize the bilingual corpus, compared to only optimized by TLM and MLM of XLM.