mSLAM: Multilingual SLAM
mSLAM stands for “Multilingual Speech and Language Model” which is a multilingual speech and language model that learns cross-lingual cross-modal representations of speech and text by pre-training jointly on large amounts of unlabeled speech and text in multiple languages. mSLAM is the multilingual version of SLAM which has been pre-trained on speech data from $51$ languages and text data from $101$ languages. mSLAM was proposed by Google in 2022 and published in their paper: “mSLAM: Massively multilingual joint pre-training for speech and text”.
On several downstream speech understanding tasks, mSLAM demonstrates significant quality improvements over equivalent models trained only on speech. When fine-tuned with only speech translation data, mSLAM is capable of zero-shot text translation in several languages, suggesting that the model is capable of learning from data in one modality to improve quality in the other.
SLAM Recap
Since mSLAM is the multilingual version of SLAM, then it makes total sense to have a quick review of SLAM. As shown in the following figure, SLAM consists of three parts: Text Encoder responsible for encoding text data, Speech Encoder responsible for encoding speech data, and Multimodal Encoder responsible for combining representations from both modalities (text & speech) into a unified representation. The main building block for these encoders is the Conformer network.
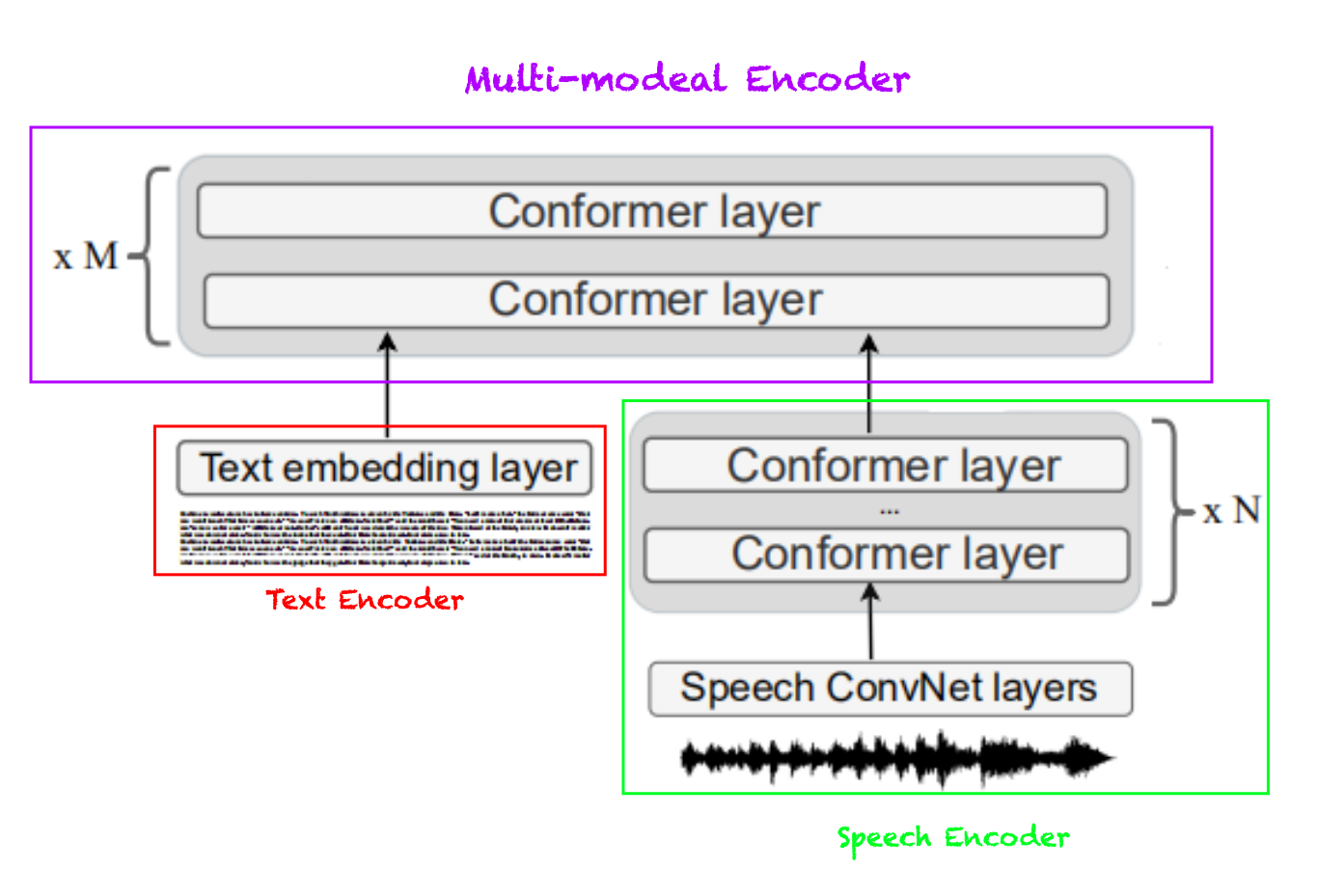
SLAM was pre-trained using multistage pre-training where it’s first pre-trained on Span Masking and Masked Speech Modeling for a few epochs ($500k\ $as mentioned in the paper), then pre-trained on TLM and SLM tasks for $250k\ :\ 500k$ additional steps. Then, the gradients of all objectives are aggregated and used to update the model parameters.
mSLAM vs SLAM
Even though mSLAM is the multilingual version of SLAM. researchers made some changes to SLAM to further improve the performance. These changes can be summarized as the following:
-
They skipped the Speech-Text-Matching (STM) task since preliminary experiments didn’t reveal any advantages Translation Language Modeling (TLM).
-
In pre-training, they used a character vocabulary, containing $4096$ tokens spanning $101$ languages, instead of using $32k$ token sentence-piece tokenization. This results in longer sequence lengths, which they capped to $512$ characters.
-
They increased the length of masked spans from $5$ to $20$ tokens for the SpanBERT objective.
-
They applied a CTC loss on the speech portion of the paired input, using the character-level transcript as the target. This CTC loss ensures stronger alignment between the speech and text representations learned by the model.
-
In the 2-Billion version of the model, they increased the model dimension from $1024$ to $1408$, and the number of of conformer layers in the Multimodal Encoder to $32$ layers instead of $16$ while keeping the conformer layers in the Speech Encoder at $8$ layers making the total conformer layers of mSLAM $40$ instead of the $24$ of SLAM. Also, the peak learning rate is reduced from $6e^{- 4}$ to $3.6e^{- 4}$ for better training stability. Other hyper-parameters remained the same.
-
During pre-training, they didn’t used multistage pre-training. Instead, they combined all modalities in the same batch as we are going to see in the “pre-training” section.
So in summary as you can see in the following figure, mSLAM is pre-trained on three different types of input data:
-
Text-only: mSLAM uses Masked Spanning task, adapted from SpanBERT, with longer span ($20$ tokens) than SLAM ($5$ tokens).
-
Audio-only: mSLAM uses Masked Speech Modeling (MSM) task, adapted from w2v-BERT.
-
Speech-text pair: mSLAM uses Translation Language Modeling (TLM), adapted from XLM, with the addition of the CTC which improved the speech-text alignment and disregarded the STM task.
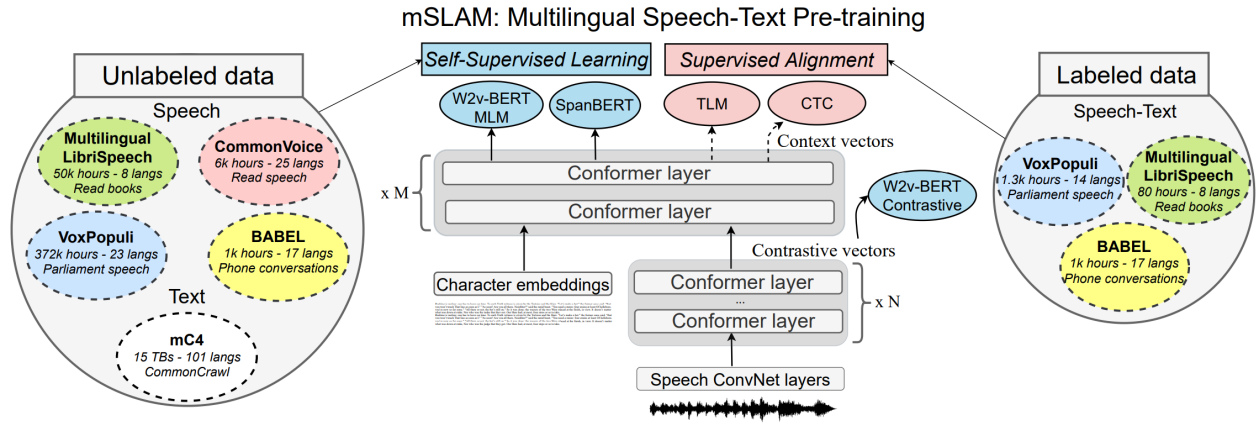
Pre-training
At each training step, they trained mSLAM on all three types of data; each batch is composed of $2048$ sequences of unlabeled speech, $8192$ sequences of text and $256$ sequences of paired data. They used the Adam optimizer with a Transformer learning rate schedule with $40k$ warmup steps, linearly increasing the learning rate to $6 \times 10^{- 4}$, followed by inverse square root decay. They trained mSLAM-base (600M parameters) for $1.3M$ steps and the mSLAM-large (2B parameters) for $350k$ steps.
The three types of data was divided into the following stats:
-
Unlabeled speech: They used approximately $429k$ hours of unlabeled speech data in 51 languages. This data is divided as:
-
$372k$ hours spanning $23$ languages from VoxPopuli.
-
$6k$ in $25$ languages drawn from CommonVoicev6.1.
-
$50k$ hours of $8$ languages from MLS (Multilingual LibriSpeech).
-
$1k$ hours of telephonic conversation data spanning $17$ African and Asian languages from BABEL.
-
-
Unlabeled text: drawn from mC4 dataset which consists of natural text in 101 languages drawn from the public Common Crawl web scraper. During pre-training, they up-sampled lower resource languages using temperature-based sampling, with $T = 3.0$.
-
Speech-text Pairs: They used approximately $2.4k$ hours of paired speech and transcript data spanning $32$ languages. This data is divided as:
-
$1.3k$ hours of speech and transcript data spanning $14$ languages from VoxPopuli. They excluded languages with less than 1 hour of data.
-
$80$ hours from MLS (Multilingual LibriSpeech). They uses the 10 hour training splits of the paired data for each of the 8 MLS languages.
-
$1k$ hours from BABEL dataset spanning 17 languages from the Babel ASR task.
-
Experiments & Results
In this part, we are going to talk about the different downstream tasks that mSLAM was fine-tuned for; and discuss the different fine-tuning configurations for each of these tasks.
Multilingual Speech-to-text Translation
For multilingual speech-to-text translation task, they attached a $6$-layer, $512$-dimension Transformer decoder to the pre-trained mSLAM model with a dropout probability $0.3$ on the input embedding and all residual connections in the Transformer decoder to mitigate overfitting. In this task, they tried two different fine-tuning setups:
-
Speech-only Finetuning:
They used the speech translation data in CoVoST2 dataset. They evaluated on a multilingual X-to-English task that covers translation from $21$ source languages into English. -
Speech + Text Finetuning:
In addition to the speech-only data from CoVoST2 dataset, they used text data from either WMT or TED Talks, as available per language. For example, they used WMT20 for “ja”, “ta”; WMT19 for “de”, “ru”, “zh”; WMT18 for “et”, “tr”; WMT17 for “lv”; WMT15 for “fr”; WMT13 and TED59 for “ar”, “fa”, “id”, “it”, “nl”, “pt”, “sl”, “sv”. While finetuning, each training batch contains equal numbers of ST and MT examples, with a higher loss weight, $5.0$, on the MT objective. A lower dropout probability $0.1$ is used as more training data is available.
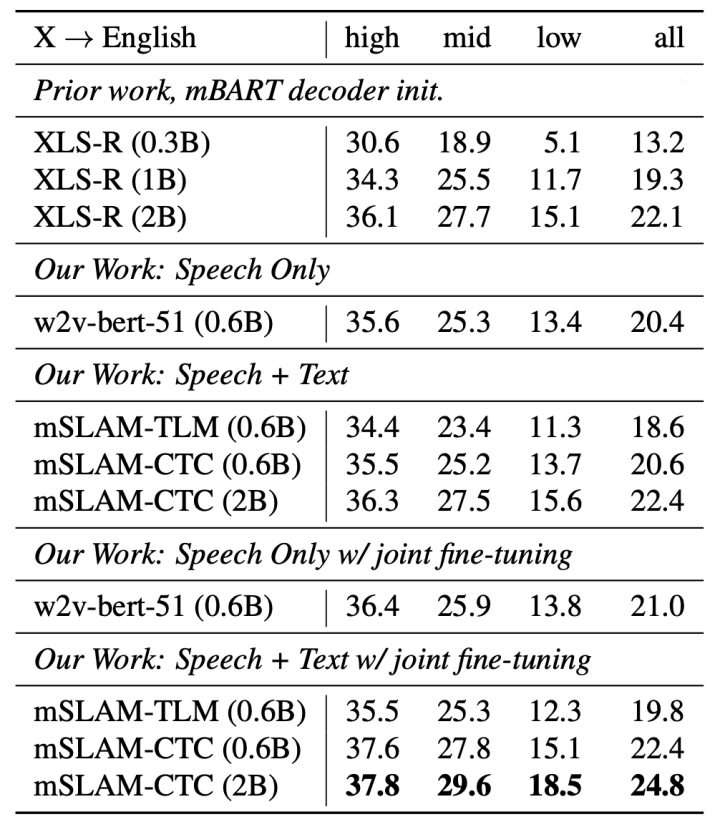
A comparison between mSLAM and other multilingual models such XLS-R and w2v-bert (trained on the speech-only data of mSLAM) is shown in the following table. As we can see, the 2B version of mSLAM with speech+text finetuning has the highest scores across all resources.
Note:
mSLAM-CTC is the version of mSLAM that was pre-trained on span masking + TLM + CTC loss function; while mSLAM-TLM is the one that was pre-trained on just span masking + TLM.
Multilingual Speech Recognition
For multilingual speech recognition task, they attached the pre-trained mSLAM with a 2-layer LSTM as a conformer-transducer model. For this task, they used a merged grapheme vocabulary based on the task-specific training set for all ASR fine-tuning experiments without the use of any language model. They fine-tuned a model in a multilingual setup of VoxPopuli + MLS. And they fine-tuned separate models per language for Babel. in finetuning, they used a peak learning rate of $3e^{- 4}$ with $5k$ warm-up steps and $1e^{- 3}$ and $1.5k$ warm-up steps for encoder and decoder respectively. All finetuning experiments on speech recognition used a constant batch size of $256$.
The results can be shown in the following table where you can find that mSLAM achieves competitive ASR results without losing speech capacity across a variety of ASR tasks and languages. Increasing mSLAM-CTC capacity to $2B$ parameters results in improvements over both, the $600M$ model and our speech-only baseline.
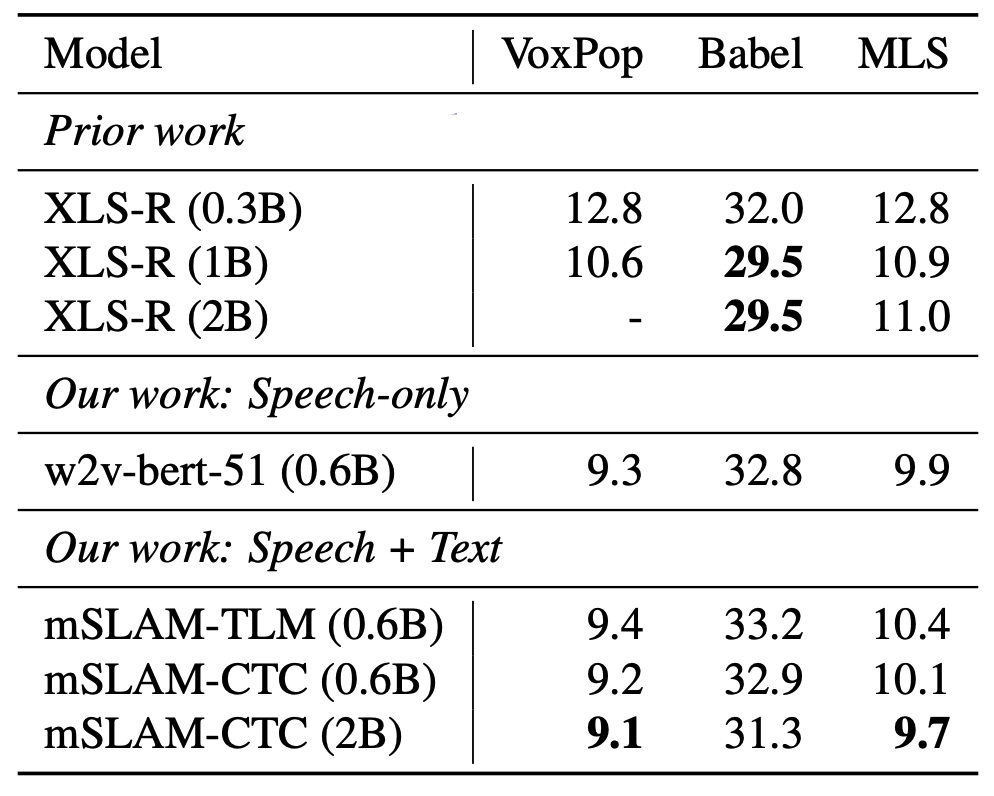